Integrating drones into vector surveillance Surveillance is defined as “the continuous and systematic collection, analysis, and interpretation of disease-specific data and the use of those data in the planning, implementation, and evaluation of public health practice” and has been recognized by the WHO as a core intervention for vector-borne disease control [47].
Surveillance A key surveillance priority is monitoring the distribution and abundance of local vector species to evaluate potential impacts on disease transmission. This requires keeping track of vector habitats and assessing changes that may increase or decrease vectorial capacity directly (e.g. landscape change that creates a suitable habitat) or indirectly (e.g. increasing availability of blood meals, resting sites or predation) or leads to ecological shifts in biting behavior or the predominant vector species [48]. Entomological surveillance is essential for monitoring and evaluating interventions, identifying priority areas for further surveillance and assessing vector-borne transmission potential, and for determining whether the migration of infected people or vectors into an area or the replacement of one vector species by another is likely to lead to disease outbreaks. Generating accurate maps of mosquito breeding sites might also provide an opportunity to use them as information, education, and communication materials to display specific risk conditions for a given locality [47].
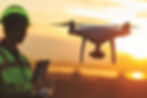
Earth observation
Earth observation (EO) is the collection of information on the physical characteristics of Earth’s surfaces using remote sensing technology, such as satellites, manned aircraft, or drones. Raster data from EO are used frequently to characterize vector habitats and to monitor changing risks [49]. EO uses sensors that measure reflectance or emitted radiation from a distance, with wavelength interval size and a number of wavelengths measured on the electromagnetic spectrum defining the spectral resolution of the sensor [50]. These sensors are also characterized by radiometric resolution, i.e. the sensitivity of the sensor to measure differences in reflected or emitted energy. While RGB sensors measure only the visible range of the electromagnetic spectrum (similar to standard cameras), sensors with a higher spectral resolution increase the capacity to detect specific habitat types and allow transformations, such as for vegetation indices. Additionally, EO data are characterized by spatial resolution, which is the size of a pixel within the image, and by temporal resolution, which is the frequency at which the data are collected. While satellite data often have much higher spectral resolution than data collected by drones, the spatial resolution of satellite data is typically more constrained because freely available data are limited to a resolution of ≥ 20 m/pixel; higher resolution data are often prohibitively expensive. In contrast, typical aerial imagery collected by drones has a resolution of < 10 cm/pixel (Fig. 3). Additionally, drones offer distinct advantages for temporal resolution as the user is able to define flight timing, whereas satellite data are only available when a satellite is passing over a specific location and may be further limited by cloud cover obscuring the target site [46]. Despite these advantages, drones cover much smaller geographical areas than satellite imagery and satellite data are more appropriate when large regions are targeted. For example, typical applications of low-cost drones result in < 1 km2 of the area being mapped per 30-min flight due to different reasons, such as battery life and legal permission from Aviation Authorities (weight, visual line of sight [VLO], speed, and maximum altitude) [46]. While the area covered will depend on local conditions, the types of drones used, and the flight height, commercially available drones are only able to cover limited geographical areas. Due to this limitation, drones are frequently best used to complement existing sources of EO data (Fig. 3). This may include drone use to conduct targeted mapping of high-priority areas identified by satellite-based EO data or, conversely, using drones to collect training data to classify satellite EO data.

Data sources and analysis methods to be used should be determined by the features to be identified and the resolution required for surveillance; for example, direct observation of larval breeding sites at set time intervals for targeted larval source management. In other cases, the objective may be monitoring landscape changes or estimating the number of people at risk or potential disease hosts. Based on these features and the technical capacity available, a specific approach to analyzing drone data should be determined. This approach can include the following steps:
Manual digitization: the simplest approach that relies on the visual identification of key features; this has been shown to be effective for pinpointing malaria vector breeding sites in Tanzania [20].
Region-growing/technology-assisted digitizing: this approach builds on manual digitization by using automated approaches to classify similar neighboring pixels [51].
Unsupervised classification: these methods group features within an image into different categories without the use of training data to define classes of interest. Such methods are used less frequently alone as they require the user to determine subsequently what the categories represent.
Supervised classification: methods that use training data to fit a model classifying the imagery into pre-defined categories of interest. Training data may include specific categories or use ground-based data for imagery analysis. An example of pixel-based supervised classification is found in the section Mapping juvenile aquatic habitats (Case study 1).
While a wide range of different models can be used for all approaches, machine learning methods are being utilized increasingly for image analysis. These can be implemented in a range of open source and commercially available software, with the additional cloud-based computing power available through online platforms such as Google Earth Engine and Amazon Earth. Additional considerations should include the technical skills of the data analyst; while manual digitization and region-growing approaches can be used by non-experts, significant technical expertise is required to implement machine learning approaches.
The features to be identified and the analytical approach should determine the selection of the drone sensor. Commercially available drone sensors include RGB, multispectral and thermal cameras, and, increasingly, a range of active sensors, such as three-dimensional (3D) laser scanning (i.e. LiDAR) (Fig. 4). To collect data, the drone, and sensor is flown over the AOI, typically in a grid-like configuration to allow the collection of overlapping images. Using commercially available (e.g. Agisoft Metashape Professional; Agisoft LLC, St. Petersburg, Russia) or open-source (e.g. Open Drone Map) software, these images can then be processed to generate spatially referenced ortho mosaics, which are images that have been stitched together and orthorectified. Additionally, photogrammetric methods (or Structure-from-Motion) can generate digital surface models and 3D representations of landscapes. These data can then be used as inputs into image analysis pathways or input directly into a Geographic Information System (GIS) software for viewing.
Drones are advantageous in their ability to detect small-sized features, as they produce high-resolution imagery at the sub-meter level. This is particularly important in vector-borne studies because water bodies/containers suitable for mosquito breeding are frequently small. The top row shows a comparison of the pixel size produced by a drone in contrast to two commonly used freely available satellite imageries. The middle row demonstrates changes in the landscape composition across a 2-month window, as captured by drones, which otherwise might be overlooked by satellite images. The bottom row shows images with common bands that are available in the sensor often used in drones: RBG cameras (left), multispectral cameras that have NIR and red edge band (middle) and an NDVI composite using red and NIR bands (right). MS, Mass spectrometry; NDVI, normalized difference vegetation index; NIR, near-infrared; RBG, red, green and blue color model
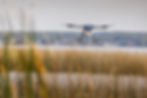
Mapping juvenile aquatic habitats
Aquatic habitats used by malaria vectors have a spatially heterogeneous distribution that is controlled mostly by hydrological and geomorphological processes [54], together with regulated land use/land cover (LU/LC) [55, 56], resulting in a patchy distribution of water bodies, which are often spatially organized in clusters [57]. The distribution of aquatic habitats is also strongly correlated with rainfall, which can dictate the temporal dynamics of anopheline larval habitats and adult abundance [58,59,60]. Mapping the spatial distribution of aquatic habitats preferred by mosquitoes is crucial to the successful deployment of different control and surveillance practices. Also, targeting the most productive habitats could increase the cost-effectiveness of mosquito larval control programs [61, 62].
Remote sensing and geospatial mapping are realistic approaches to identifying larval habitats that cover large areas, overcoming some operational challenges of conventional ground-based surveys ]. The identification of larval habitats using high spatial resolution satellite imagery may be challenging during the classification process, particularly when water bodies are small and turbidity is high [66, 67]. Another example of the use of remote sensing technology is malaria risk assessments through the study of anopheline larvae [68, 69]. Recently, object-oriented machine learning classification has been explored to overcome backscatter similarities of radar data when mapping open water or slightly vegetated areas between stretches of open water [70]. Proof-of-concept studies have proven the feasibility of using drones to map aquatic larval habitats of African [20, 71] and South American malaria vectors [22] (Table 1). The high-resolution imagery at a relatively low cost compared with satellite images, the temporal flexibility to capture images by the drone user, and the clear-sky conditions required for optical satellite imagery make this technology an effective operational tool for the identification of potential habitats [46] (Case study 2; Fig. 5).
Table 1 Examples of the use of uncrewed aerial vehicles in vector-borne diseases showing ecosystems, the purpose of the study, mosquito species targeted, and technical characteristics of the drone surveys.
Deforestation disrupts wildlife habitats and can bring human, mosquito, and macaque populations in closer proximity, thereby increasing the potential for disease transmission. Monitoring wildlife populations is essential for understanding disease dynamics in these changing landscapes. b Inspection with thermal cameras The proliferation of larval habitats of anopheline malaria vectors has been also associated with human activities, such as agriculture, settlement, mining or other landscape alterations that incorporate deforestation and vegetation clearance [72,73,74,75]. More frequent surveillance via drones will allow for the detection and subsequent development of quick responses to rapid changes and environmental alterations, such as urbanization, or changes in the use of rural/agricultural areas that might lead to new aquatic habitats favored by certain mosquito species. Aedes-borne disease surveillance programs, such as those for dengue, chikungunya or Zika, commonly use periodic household inspections for detecting water-holding containers positive for Aedes larvae [76, 77]. Estimation of infestation indices, used to define and inform control actions, are labor-intensive in terms of vector intervention and public health staff and can be inaccurate due to variation in searching efforts, householder availability, and/or mosquito egg-laying behavior; they may also be intrusive for residents. The centimeter-scale of the preferred oviposition habitats of Ae. aegypti and Ae. albopictus precludes the possibility of using high-resolution satellite imagery typically used for monitoring large water bodies for malaria vectors [20]. Approaches that leverage the high-pixel resolution of drone imagery have been explored to identify and map potential breeding sites characteristic of Aedes, such as cisterns, pots, tyres or flower pots. Ultimately, these approaches might support specific vector strategies, such as targeted application of larvicides against Ae. aegypti in, for example, rural areas of Central America [78] or guide preventative actions and prioritize programs that focus on controlling this species (e.g. in the city of Cuiabá, Brazil [79]). In urban areas, drone imagery has also been used to locate preferred oviposition sites of Ae. albopictus [36]. In a study combining aerial imagery and entomological surveys in New York State (USA), up to 64% of the containers with water identified in ground surveys were detected with high-pixel resolution images and 14% were partially visible from the air (Table 1). In this suburban setting, the detection of containers depended on the location within the property, with difficulty viewing those located on porches, trees, underneath awnings or near walls. Visibility was also affected by common features, such as tree cover and the location of the drone when taking pictures [36]. Case study 1: Carrasco-Escobar et al. 2019 [22] Carrasco-Escobar et al. [22] used drones mounted with regular RGB and multispectral cameras to collect high-resolution images to determine the spectral signature (characteristics of the light reflected from the land/water surface) of the most productive breeding sites of the malaria vector Ny. darlingi nearest to human habitation in Amazonian Peru. These researchers conducted a supervised classification approach (overall pixel-based accuracy: 86.73–96.98%) to identify water bodies and Ny. darling-positive and -negative areas within the water bodies were identified, respectively, using machine learning techniques. They used a two-step process involving first the classification of several land type coverages and second the masking and classifying of only water bodies. The methodology and analysis were proofs-of-concept for testing whether improved larval source management can be combined successfully with LLINs and IRS to contribute to the elimination of transmission in malaria hotspots in the Amazon. Case study 2: Stark et al. 2019 [80], Jumail et al. 2021 [81] In addition to collecting data on mosquito habitats, drones are valuable for monitoring wider environmental factors influencing disease dynamics. Vector-borne diseases such as Plasmodium knowlesi and yellow fever are maintained by wild non-human primate reservoirs. Drones enable the mapping of fine-scale deforestation and other habitat modifications that influence the movement patterns of wildlife populations [80]. Specifically, Jumail et al. [81] explored the use of thermal imagery to capture heat signatures of wild animals and estimate, in real-time, wild primate populations within changing landscapes in the Lower Kinabatangan Wildlife Sanctuary in Malaysian Borneo [81, 82]. These methods were validated initially by ground-based surveys prior to deploying aerial thermal surveys, with the objective of developing non-invasive techniques to assess wildlife populations and study disease dynamics.
Vector control As discussed in the previous section, drone application in mosquito vector control has been related mainly to larval source management (LSM). This strategy seeks to reduce the immature stages of malaria vectors using habitat modification or manipulation, larviciding or biological control [83]. When implemented well and incorporated into ongoing vector intervention programs, LSM can reduce the densities of indoor and outdoor biting mosquitoes and help decrease the dependence on insecticides, and therefore help prevent the emergence of insecticide-resistant mosquitoes. However, WHO recommends larviciding only for special cases “when breeding sites are few, fixed and findable, and where the sites are easy to identify, map and treat” [62]. Such landscapes are uncommon not only in Africa but also in other malaria-endemic regions like Southeast Asia and South America. The use of drones in vector control can be divided into two main applications. The first is the use of the imagery to retrieve data that can serve as (i) a source of visual inspection carried out before and after interventions; and (ii) as a communication tool, to detect water bodies and positive breeding sites and to create detailed maps of the mosquito breeding habitats and landscape. The second application is that drones can be used to disperse the intervention; for example, the dispersal of larvicide in the liquid or granular form [84] and, more recently, as a potential means of deploying genetically modified mosquitoes [85]. For these activities, both the limited UAV flight time, which is largely dependent on battery capacity, and payload can be limiting factors [86, 87]. For instance, UAVs are especially effective in spraying when they are over water (e.g. rice paddies), on irregular or sloping ground, in small areas, and in places where it is hard to reach with other kinds of equipment. However, in units > 50 Ha, conventional ground and aerial sprayers proved to be more efficient [88]. Regarding flight time, the duration is even further shortened when the drones are fully loaded. Using a network of communicating drones, or swarms, could be one approach to overcome this operational challenge [89]. Some attempts have been made to use drones to deploy larvicides by leveraging the development of drones to apply pesticides for agricultural use for example, the dispersal of a biological control product to reduce juvenile malaria vectors in irrigated rice fields in East Africa [91] or as implemented in some mosquito control programs for treating remote pools of standing water in the brackish salt marsh with larvicides (Florida Keys Mosquito Control District). Using drones as deployment devices may overcome the challenges of treating and accessing large areas on foot and make aerial distribution affordable. Also, multispectral imagery collected with commercial drones has helped to predict the operational efficacy of some larvicide formulations against Aedes vigilax in complex environments with irregular canopy cover, such as mangroves [84]. In drone spraying operations, flight parameters might influence the effectiveness of droplet deposition when using liquid products and might need adjustments according to the larval habitat characteristics [92]. Flight height and velocity have a remarkable influence on the deposition amount [93]; high-speed rotation of the rotor—and of the air—rotor wind field [94]—and the downwash airflow may also affect intervention efficiency. Importantly, the use of drones in targeted areas could reduce harm to the environment by limiting wildlife disturbance in sensitive habitats
Release of adult mosquitoes Vector control approaches built on sterile insect techniques and Wolbachia-based strategies rely on mass-rearing and the dispersal of thousands of mosquitoes at a specific frequency depending on the nature of the strategy [97, 98]. Critical operational aspects need to be addressed before the deployment and scale-up of these interventions in the areas to be treated, such as aerial coverage, operational costs associated with the number of release sites, and mosquito transportation [85, 99]. Operational programs can benefit from using drones during the implementation of these interventions and to optimize some of the key technical aspects, including remote preparation of the material and then expanding release and dispersion areas. The World Mosquito Program (WMP) has employed drones to optimize the release of Wolbachia-carrying mosquitoes for control of the dengue virus on the island of Fiji, South Pacific. There, the development of an aerial release mechanism that can store up to 160,000 mosquitoes and release 200 every 50 m has proved to be much faster and more homogeneous than ground releases and also provided better coverage [100]. In another epidemiological scenario, in the fight against dengue and Zika in Brazil, Bouyer et al. [85] designed a mechanical compartment for dispersing sterile males by using drones. This study demonstrated minimum damage to mosquitoes and similar competitiveness between those released on the ground and by drone. Moreover, this drone-based dispersion modality was estimated to provide a 20-fold reduction in the estimated costs of implementing the sterile insect technique (SIT).
Challenges and technological developments The drone industry is expanding and gaining increasing interest from a wide range of stakeholders for use in civil applications [101]. As discussed above, important developments in the industry have been focussing on safety improvements. In this section, we detail additional challenges to drone operation, as well as recent technological developments for the control and surveillance of vector-borne diseases.
Capacity building
Most countries require operator training for authorization of drone operations. However, there is still no consensus on the minimum levels of training ensuring safe flight and ground operations [102]. Despite algorithms that have been developed recently to automate common human tasks related to drone operations, overtrust, and automation bias in autonomous systems could be extremely dangerous in safety–critical settings [103]. Recently, Kucherov et al. [104] proposed and evaluated a training process for specialists in the maintenance and operation of UAVs; however, this field is still under development.
The accuracy of supervised image classification to detect breeding sites is largely dependent on the availability of training data, i.e. identified data of the features of interest used to fit predictive models [105, 106]. Recent initiatives have focussed on developing large training datasets of such features, including roads, housing, or agricultural land types [107, 108]. These datasets are typically labeled using standardized methodologies, such as the Spatiotemporal Asset Catalog [109], enabling utilization by different platforms for various purposes. However, there is no standard image repository to share mosquito habitat training data, and most projects need to collect their own. Energy management One important challenge of drone missions is the current trade-off between battery weight and flight duration, as increased weight limits the flight duration. To cover large areas, multiple returns to the charging station are needed, a potential problem for drone operations in rural areas. Current developments in wireless charging [110, 111] and lightweight solar-powered battery components [112] may improve drone operations.
Evaluation of performance There is a critical need to evaluate the impact of drones on the control or surveillance of vector-borne diseases using metrics appropriate for the intended end use. While model validation is an important step of image analysis, new approaches are needed to assess impacts on disease transmission and the efficacy of control programs. This may involve identifying target levels of sensitivity and specificity for detecting or treating specific features, similar to practices used to evaluate diagnostic methods.
Conclusions In areas with the endemic and residual transmission of vector-borne diseases, effective vector control measures must be considered for moving towards elimination or, at the very least, a dramatic reduction of pathogen transmission. A major consideration in this context, for malaria control, is targeting outdoor transmission through the identification, surveillance, and treatment of aquatic habitats favorable for juvenile vector mosquito stages. Recent developments in drones (or UAVs) can be effective for accurately conducting surveillance, assessing habitat suitability for larval and/or adult mosquitoes, and implementing interventions. Examples that leverage this technology include high-resolution mapping of water bodies in endemic areas in Latin America, Southeast Asia, and Africa. The implementation of this technology for current control activities requires specific considerations in terms of local regulations, safety, privacy, and community acceptance. In this rapidly evolving field, emerging technological developments are addressing these concerns. However, despite the rapidly increasing availability of this technology, drones remain best used in conjunction with other existing approaches, such as field surveys, analysis of satellite-based EO data, and deployment of control measures. Drones have the potential to improve and target these existing activities, adding to a growing suite of tools for vector control.